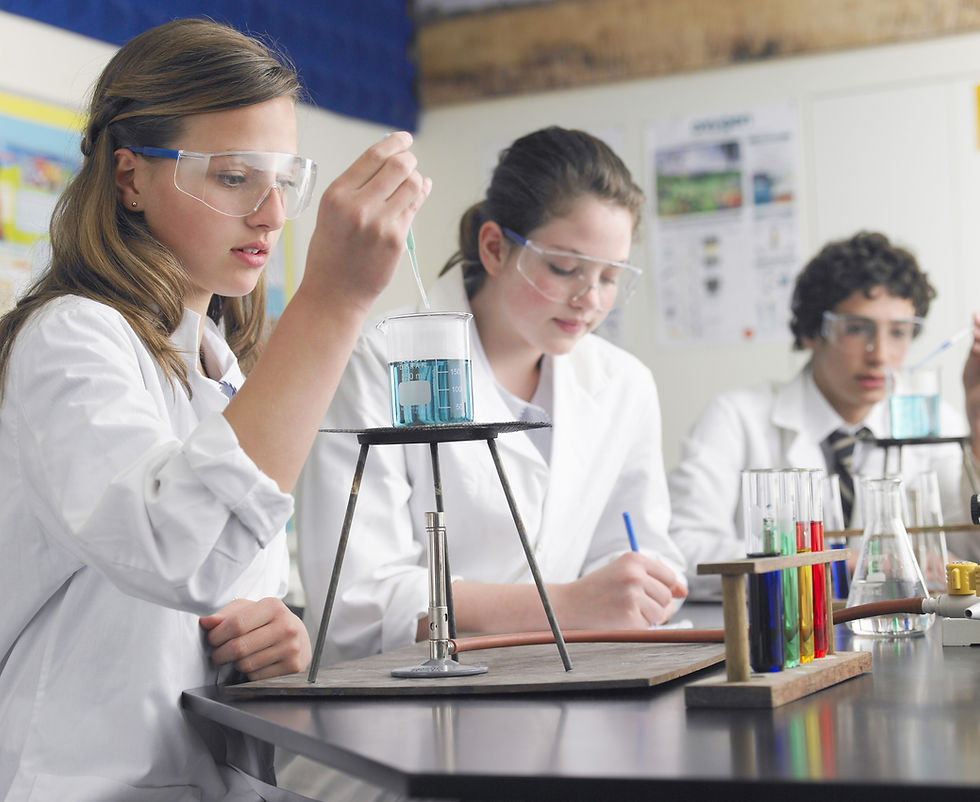
I have recently been fascinated with the data analytics concept. I have done some research and it lead me to few great insights that are fundamental to changing my approach to data analytics.
Two main trends emerged within businesses analytics recently.
1) Behavioural science - with increased awareness of cognitive biases. The big idea is that we can better understand how markets and customers work if we better understand human behaviour.
2) Computer science - with increased availability to store and analyse data. Machines allow to outperform human tasks more and more. It also involve the science of computers being able to save the problems of human psychology.
However, this all is not that simple. The question is: what does it means to be data driven in practice? One could say that analytics programs should be developed and company investments should be made in data driven environments. Is that the solution?
One very simple answer to all the confusions, is that we should not allow computer to answer all the data questions.
We use psychology.
Data-driven environments
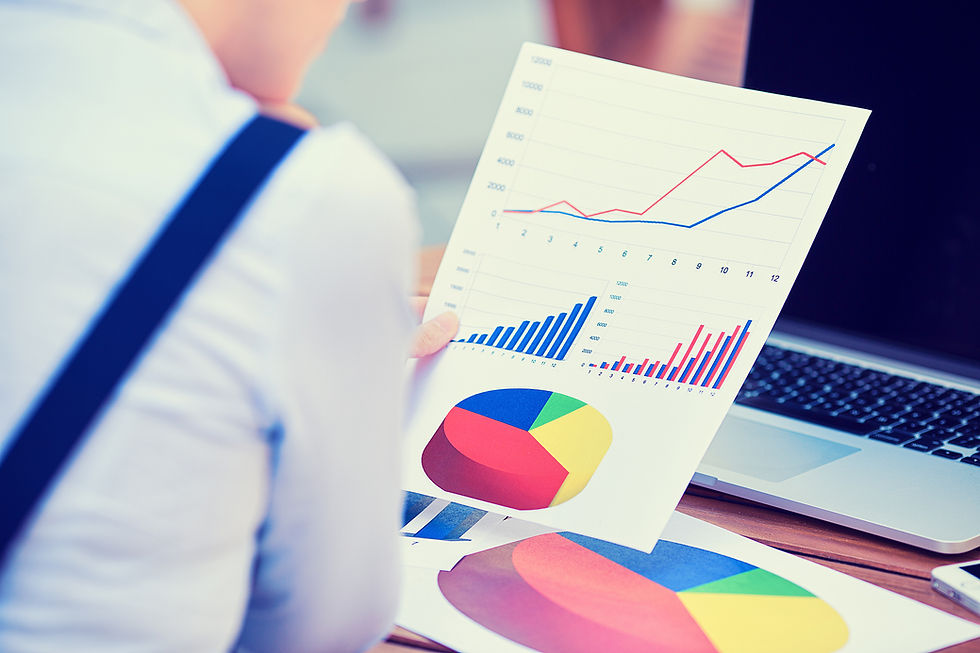
Data-driven approaches in itself can miss the critical thinking process when evaluating the data. By using only data as a primary source on taking action is not enough. There need to be an element of analysis and critical thinking involved by human-being beforehand. Another step of making a decision what other data may be needed to take actions first.
This is because, the data structures are not always configured to predict and determine be human behaviour correctly. Data on its own does not often consider the true impact or effectiveness of interventions to be made and and can lead us to taking incorrect actions.
Sometimes data answers the wrong questions.
Companies increasingly adjusting their data to help their decisions making. But at times companies don't understand what their data is showing them. The company needs deeper insights into data. For example the customer priorities and target the interventions to those who are most likely to be positively affected by any manipulations.
Before we take actions based on data here, we need to understand how likely the customer is to react and what is the effect of intervention on this customer likelihood to react.
At times, test and experiment is required to further measure the responses in order to make better decisions.
You need to run experiments before interventions to better predict the response behaviour.
Actions should not be data-driven, it should be decision-driven. We can start from thinking "wide-to narrow". This means that, when evaluating data we should start will as many available options as possible and narrow them down. Than, we think what data is needed further to rank the course of action.
Often, small good data is better than a lot un unuseful data. Than, we select the best point of action. Start from what is unknown. The decision driven analytics consider the leaders who need to make the decisions. In data-driven environments the focus is on the data, not the decision making analytics.
Should we be taking decisions from the data algorithms or should be take a different approach?
To make effective decisions with data and people who can handle it: statistics, mathematics etc. what we also need are people who not necessarily know the details of the programming, but have a good quality of intuition within analytics. There is a good mixture and difference between thinking and data.
Decision-driven environments

The type of thinking that need to be develop are consider to be not memorising the data, but have an idea put it apart and put it back together, being creative. Computers and data cannot also predicts the questions why.
People can do it, and people are needed to answer the questions why things could happen.
At times working from historical data become the dominant mentality in the organisations. But there is a shift needed for looking for new data and approaches. The key here is the distinction between the data-driven and decision-driven approach. It is proposed that the analitycs should be decision-driven.
First we identify the problem and after looking for the data we need. That requires deep thinking and making structures to getting the data. As opposed to first looking at the data and than making decisions. This approach will allow to determine the experiment type required to further analyse the situation.
But experiments can be costly. What types of decisions should be elevated when entering the experiment zone? Experiments should be risk-based. With experiment you can get close enough to get to the decision on relevant intervention. Experimenting can give us good insights. Thinking carefully what may lead to the best intervention can get us far with the decision on actions to take for the experiment.
Executives often push back on this new ideas at the moment. How do we overcome this? This is where we look at the benefits of decision-driven analytics. The point is find whats available out there than find data that will support it.
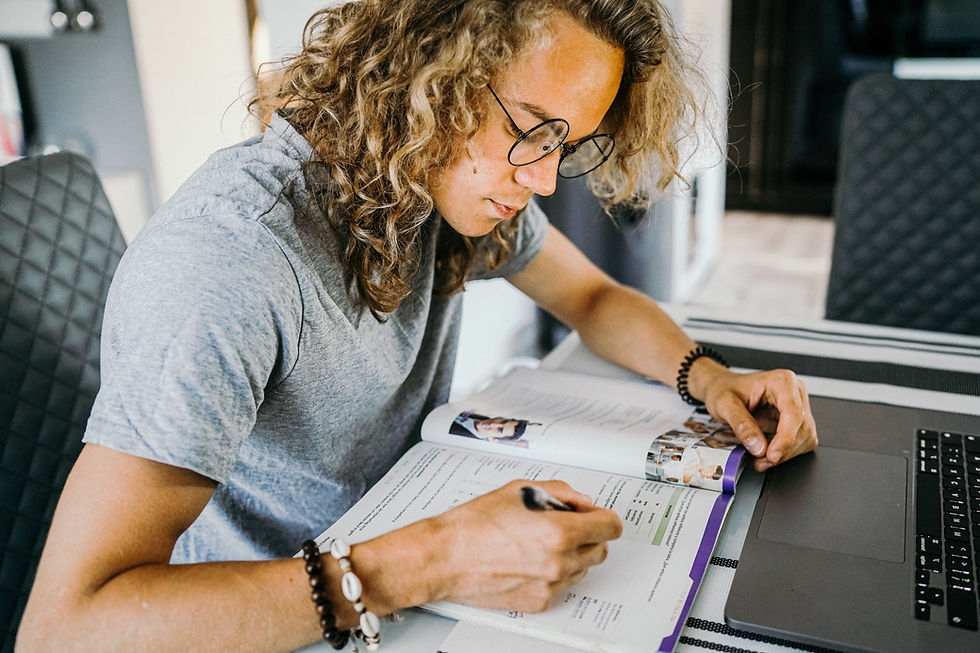
So, is the new methodology to data-analityics the way forward? There is certainly a great amount of theory that this will be the future of analytics. But there isn't enough evidence that these bring the results and not enough guidance on how this could be managed in most efficient way. My only conclusion at this stage, would be to start developing the critical thinking skills. Developing the skills of being able to widen the problems first, and from there, narrow down and drill the data required afterwards. This is certainly a fascinating new concept and has a lot of potential.
Comentários